- Plenty of Room
- Posts
- AI Protein Design: Text-Based Generation of Custom Proteins
AI Protein Design: Text-Based Generation of Custom Proteins
Plus: superlattices and more!
Welcome to Plenty of Room!
It’s time for another deep dive into the world of AI-powered protein design! This article was suggested from a subscriber: if you also have a cool paper, don’t hesitate to send it this way! Let’s jump into it now.
Plenty of Room is your guide to AI-driven protein design, DNA nanotech and more.
Love this issue? Spread the knowledge and share with your fellow science enthusiasts!
Let’s dive right in.
AI Chat for Protein Design
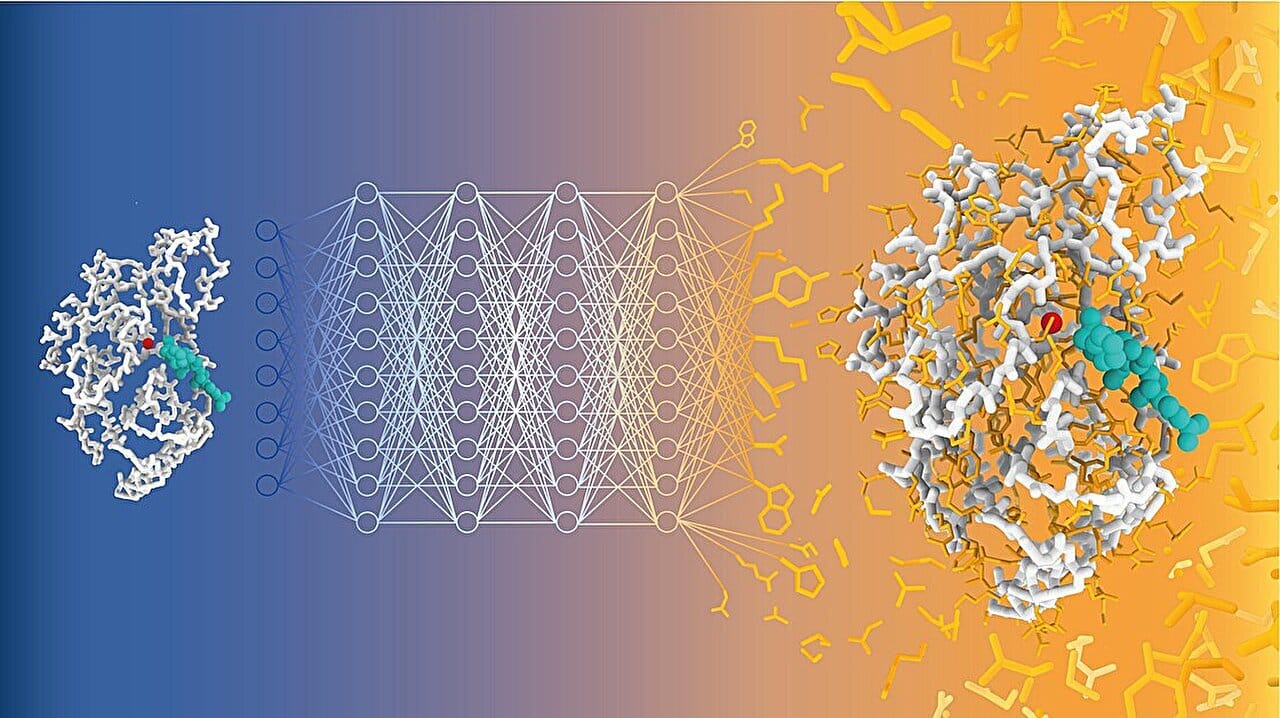
AI can create protein sequences from text prompts using ProteinDT, a new multimodal AI model. Image credit: Alexandra Banbanaste (EPFL)
We all know that proteins are one of the building blocks of life, and designing custom proteins with enhanced stability, catalytic activity, or specificity is a key challenge in synthetic biology, drug discovery and biotechnology. But we have seen a thousand time how hard and time-consuming traditional protein design is.
Machine Learning and Proteins: a Story in the Making
Machine learning and AI are revolutionizing biological research, and doing particularly well when it comes to proteins. From designing new binders and enzymes to predicting protein structures and optimizing docking, AI is accelerating breakthroughs at an amazing speed! But there are still gaps to fill:
Traditional protein design method rely on sequence and structures: they often lack info about the functions of the proteins
At the same time, biological databases like UniProt are goldmines for protein descriptions: function, stability and interactions! But these information is not used for generative designs
Last but not least, AI models have shown success with multimodal learning, which combines different data types, such as text and images (think of using AI to generate an image). Could something similar be done for proteins, combining text and sequences?
ProteinDT: AI Meets Text and Protein Sequences
The author’s of today’s paper addressed these gaps by creating ProteinDT: a multimodal framework that aligns protein sequences and text description, enabling text-to-protein generation, retrieval and editing!
The Three Core Components
ProteinDT consists of three core components:
ProteinCLAP
First, ProteinDT aligns protein sequences and their textual descriptions into a common representation (a numerical encoding, for those like me unfamiliar with AI). The researchers used contrastive learning, a technique where data points are contrasted with each other to teach a model which are similar and which are different. For example, let’s say that the researchers had a protein sequence on one side and the text “belongs to the eS17 family” on the other. The model learnt how the text and the protein sequence were related, and started to build representations containing knowledge from both text and protein sequences!
ProteinFacilitator
Now that there is a shared text-protein space, the next step is to map text inputs to protein sequences. ProteinFacilitator maps a text prompt (“A protein that is stable”) to a corresponding protein representation, which contains the information from the given text and protein sequence patterns.
Protein Decoder
Last but not least, the final stage in ProteinDT involves decoding the representation into a functional protein sequence. The researchers used not one, but two approaches here: and they both created biologically meaningful proteins!
A Brand-New Protein-Text Dataset
Before we jump to the researcher’s (impressive) results, we have to talk about one little detail: where did they get the data? Well, they built their own dataset. They mined SwissProt, a protein database with sequences and plenty of information in text form: functions, domains, subcellular localizations, modifications and more. SwissProt is the manually curated branch of UniProt, and it has extremely high quality data! And the most amazing part: they made the dataset public.
The Results: Did It Work?
Of course it did, I wouldn’t be talking about it otherwise (biases and all). But what did they actually test?
Zero-shot text-to-protein generation: Very cool name, that means generating a completely new protein sequence from a text prompt describing functions or stability. Amazing! The proteins were evaluated for biochemical validity, similarity to real proteins, and secondary structure composition. ProteinDT did great here: the model generated sequences aligned with the text description over 90% of the cases!
Text-guided protein editing: ProteinDT successfully modified existing proteins based on text input, without requiring massive labeled datasets. This makes rational protein engineering faster and more accessible. And now, when you tell your protein it should be more stable, it might listen!
Protein property prediction: ProteinDT learns representations of proteins, and they can be used to predict protein properties, such as:
Prediction of stability
Fluorescence activity
Homology prediction
Contact prediction
The model performed on par with state-of-the-art deep learning models, demonstrating that the text-sequence embeddings capture relevant biological features!
Why ProteinDT is Cool (and Limitations)
ProteinDT is the first model to integrate text-based functional descriptions into protein sequence generation. It’s not the first text-based protein design software (I had fun with this one at 310.ai) but the previous ones mostly rely on structural data. These are great, but:
Structural data is limited. Large experimental datasets exist for only a few protein functions, and generating more is costly and time-consuming.
Function-specific models require labeled data. Specialized AI models often need function-specific experimental datasets, which are hard to obtain.
This is where ProteinDT can help jump start the process, by leveraging existing high quality text data! And it doesn’t need massive labeled datasets.
At the same, the authors highlight 2 limitations of their work:
Dataset size: SwissProt only contains around 440,000 protein-text pairs, which is small compared to other AI training datasets. This is because of the manual curation of SwissProt: the data are of the highest quality, but the process is slow. A possible solution could be mining literature for more data, but that comes with lower quality (reproducibility crisis and all that),
Evaluation: Testing proteins is expensive and hard, especially for some property. This is why here the model was evaluated only in silico. While the evaluations were robust, real-world testing is still needed!
Applications: where would you use it?
Even with these limitations, ProteinDT makes design much easier, and this has broad applications:
Drug discovery: Rapid protein engineering based on desired drug properties.
Synthetic biology: Design of novel enzymes and metabolic pathways.
Protein therapeutics: Engineering stability, binding affinity, and immunogenicity.
This paper was amazing! Cool, in depth but at the same time extremely well explained! I think they did a better job than me at explaining (no I am joking): just go and read the paper for yourself here!
And as always, thank you for reading! What are your thoughts on this paper? What would you use it? What has you most excited? Reply and let me know!
More Room:
Superlattices of DNA origami: It’s never a bad idea to mix DNA origami and nanoparticles. And it’s even better when the scale gets big. This study achieves 2D chiral superlattices of nanoparticles using DNA origami, overcoming a key challenge in colloidal crystal engineering. By spreading a DNA origami array on a substrate and attaching DNA-encoded metal nanoparticles at precise positions, researchers created large-area chiral patterns. The study reveals that local plasmonic couplings drive the superlattices' optical properties. This breakthrough enables new advancements in metamaterials, photonics, and optoelectronics.
Studying messy DNA origami: Moving from disorder to order is very important in biology, and even more in self-assembled materials. This study introduces a strategy to induce disorder-to-order transitions in DNA origami, inspired by intrinsically disordered proteins. Using a triangular DNA origami model, researchers categorized DNA staples into three subsets, each leading to metastable, disordered structures with high energy fluctuations. When the remaining staples were added, these structures self-assembled into ordered triangles within two hours at room temperature, with yields reaching 60%. This approach demonstrates a controlled folding pathway, offering a promising method for engineering biomimetic DNA molecular machines.
Designed peptide nanopores: There are plenty of protein nanopores, but most of them are big and bulky. This study designs and characterizes transmembrane peptide channels using coiled coil-based α-helical barrels. In lipid bilayers, these peptides exhibit two conductance states: one linked to sequence-specific barrel geometries and another with dynamic, large channels similar to natural barrel-stave peptides. The findings provide a framework for designing tunable membrane-spanning channels.
Not yet a subscriber to Plenty of Room? Sign up today — it’s free!
You think a friend or a colleague might enjoy reading this? Don’t hesitate to share it with them!
Have a tip or story idea you want to share? Email me — I’d love to hear from you!
You have something you would love me to cover? Just reach out here or on my social!